Putting the ‘science’ in political science
Faculty of Science researchers develop data visualization tool to analyze speech patterns in the 2016 U.S. presidential debates
October 28, 2016
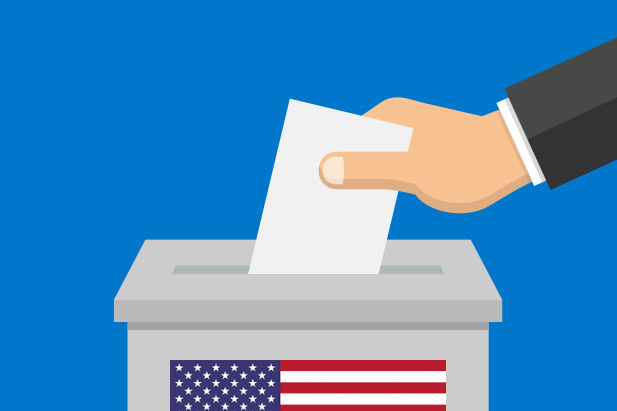
In the weeks leading up to the November 8 United States election, political experts are closely examining the leading candidates’ words in an attempt to provide some guidance for voters, who now face an important decision. Some scholars will take a word-analysis approach to the three televised presidential debates, poring over Hillary Clinton’s and Donald Trump’s utterances, watching for phrase repetitions, unique words and complexity of speech, all of which shed light on each candidate’s strategies, priorities, values and personality traits.
Naturally, there’s a sea of text data that needs to be examined before any conclusions can be drawn. While computer-assisted methods are playing an increasingly important role in fulfilling this task, until now most of these methods have focused on either revealing higher-level themes or delving into the details of the text.
Computer Science researchers at the University of Ontario Institute of Technology (UOIT) have collaborated with scholars at the University of Konstanz, Germany to develop a new textual analysis technique: one that automatically analyzes argumentation patterns in multi-party conversations and presents the data in a visual format. Mennatallah El-Assady, the University of Konstanz PhD student leading the work, is co-supervised by UOIT’s Dr. Christopher Collins, Canada Research Chair in Linguistic Information Visualization.
Known as the VisArgue project, El-Assady has combined the University of Konstanz’s visual text analytics research with the work happening at Dr. Collins’ Visualization for Information Analysis Lab (vialab) to create a tool that allows analysts to discover broad thematic patterns as well as conduct a detailed inspection of the content—based only on a transcript of the debate.
To produce a high-level overview of the debate, the team uses Lexical Episode Plots, a visualization technique that identifies word-chains (phrases or terms) that appear more frequently than an average phrase would appear throughout the text.
Delving deeper, the visualizations provide insight into the relationship between different concepts mentioned in the debate—a sort of ‘mind map’ of what the speakers brought onto the conversation floor. For example, in a visualization of the first debate you can spot a cluster of keywords such as “income,” “tax” and “audit” that represent Clinton’s call on Trump to release his tax returns.
The tool’s Conversation-Topic-Visualization function reconstructs how speakers interacted, how many topics they covered in total, and which topics they focused on.
The topic-space view from the first debate shows Trump contributing to a wider variety of topics than Clinton. At first glance, this might suggest Trump made a larger number of substantial comments than Clinton. But as UOIT Political Science researcher Dr. Alyson King points out, the two candidates have two very different rhetorical styles, with Trump being highly repetitive and Clinton using more varied language. Just because Trump used a certain word several times or covered many topics, doesn’t necessarily mean he said more than Clinton.
“Looking at the topic-space views, it might appear that Trump spoke more broadly on a variety of topics and Clinton did not; yet, if you watched the actual debate, Trump has a tendency to not complete his thoughts, and to repeat words without actually saying much that is substantive,” explains Dr. King, Assistant Professor, Faculty of Social Science and Humanities (FSSH). “He appeared to be more scattershot in his style, jumping from one topic to another without always connecting his ideas. Clinton’s style is more direct and precise, and she is less repetitive in her wording. This difference in style seems to translate into the wider path within the circle. At a surface level, the wider path trajectory associated with Trump’s utterances might seem better or positive, and Clinton’s narrower path might appear negative - but in the context of what was actually happening in the debate, the opposite might be true.”
While visualizations generated by computer algorithms might not be able to fully replace political analysis done the old-fashioned way, they do represent a fruitful opportunity for cross-faculty collaboration between computer and social science researchers.
“Big-data aggregation and visualization techniques are well established, but scholars are still working on how to make that glut of data mean something, socially and politically,” says Dr. Tanner Mirrlees, Assistant Professor, FSSH, whose research interests include political communication. “Vialab’s joint work with the University of Konstanz highlights an opportunity to bridge the gap between science, technology, engineering and math scholars who research, develop and operationalize new data visualization techniques, and social science and humanities scholars who are trained to interpret, give meaning to and make value-judgements about the data.”
- Read the full report: Visual Analysis and Exploration of the 2016 US Presidential Debates