Faculty of Science researchers get real on artificial intelligence
Hendrick de Haan, Faisal Qureshi and Martin Magill exploring the complex relationship between math and machine learning
September 17, 2019
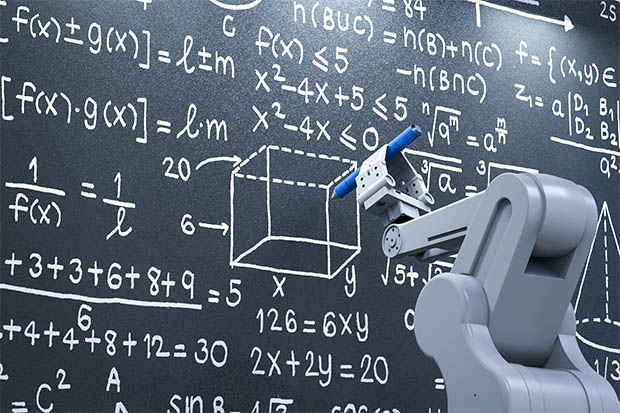
Programming a computer is a bit like teaching it how to bake: you give the computer a recipe, and the computer takes care of the rest of the work.
But beyond sheer mathematical calculations, can computers make the same subjective decisions that a human might make: such as a medical diagnosis, a treatment plan proposal, or even an investment or trading decision?
The exploration of how machines can learn by learning from human examples is a fast-growing area of academic research. In the realm of ‘machine learning’, the goal is to let computers figure out those programming recipes all by themselves. Perhaps computers will ultimately be capable of solving ideas too complex for the human mind to contemplate, let alone write down. What would that mean for society?
One very popular approach to machine learning is to equip computers with mathematical tools inspired by biological brains. Known as ‘deep neural networks’, these tools have been astonishingly successful at teaching computers how to see, speak and think like humans. In fact, deep neural networks work so well that it can be challenging to understand why they are doing what they are doing—even for their human creators.
At Ontario Tech University, Faculty of Science researchers Dr. Hendrick de Haan and Dr. Faisal Qureshi, along with Modelling and Computational Science PhD candidate Martin Magill, dissect these artificial brains in what they call their ‘mathematical laboratory’.
“One of the problems in understanding, for instance, how a computer sees a cat, is that we don’t even fully understand how a human being sees a cat,” says Martin Magill. “Instead of tackling that kind of problem directly, we decided to study how deep neural networks learn to solve very specific mathematical ‘puzzles’. In calculus, we call these ‘partial differential equations’. We know exactly how these puzzles work, so it’s a lot easier to decipher how the connections inside the network have learned a lesson. Once we know what the network is thinking, we can apply our tools to help explain how computers understand images, videos or language.”
These mathematical models or puzzles commonly create computer simulations for many important technological devices such as cars, planes, wind turbines or even nuclear reactors. Understanding why and how a neural network thinks is important in many applications.
“Without knowing the reasoning behind a network’s predictions, it is difficult to trust its judgment,” says Magill. “If a neural network recommends a dangerous course of treatment for a medical patient, for example, it would be much better to understand why the network thinks the risky treatment is justified. Or, in the world of finance, some investments cannot be made without clear justifications on why a specific investment is reasonably safe in terms of risk.”
The research by de Haan, Qureshi and Magill demonstrates neural networks can provide a new kind of information about these complex math models--information that can inform humans as to why a device is performing a certain way.
“Partial differential equations are very powerful modelling tools for studying the world,” says Magill. “Neural networks seem to have a unique capability for solving very high-dimensional equations, which often can’t be solved with today’s cutting-edge techniques. We wanted to know how neural networks succeed where other ‘traditional’ methods fail.”
Those methods have to consider every combination of a given problem’s dimensions.
“The cost of doing so grows exponentially in higher dimensions—this is known as the ‘curse of dimensionality’,” says Magill. “We suspected the key might lie in the networks’ ability to automatically break a difficult problem into smaller, meaningful pieces. This type of behaviour was already seen in networks that learned to see like humans; for example, they learn to build pictures of cats out of colours, shapes and textures. We showed that neural networks do something similar when they learn to solve differential equations. Whereas traditional methods only tell you what the answer is, neural networks can also tell you something about why that is the answer.”
In December 2018, the researchers presented their latest findings at the Conference on Neural Information Processing Systems (NeurIPS) in Montreal, Quebec. More than 8,000 delegates attended, making it the world’s largest research gathering on artificial intelligence. Of the nearly 5,000 new research papers submitted to NeurIPS in 2018, only 20 per cent were accepted, including the Ontario Tech University paper offering a new recipe on the study of neural networks that solve partial differential equations.
Media contact
Bryan Oliver
Communications and Marketing
Ontario Tech University
905.721.8668 ext. 6709
289,928.3653
bryan.oliver@ontariotechu.ca